Thinking with Data
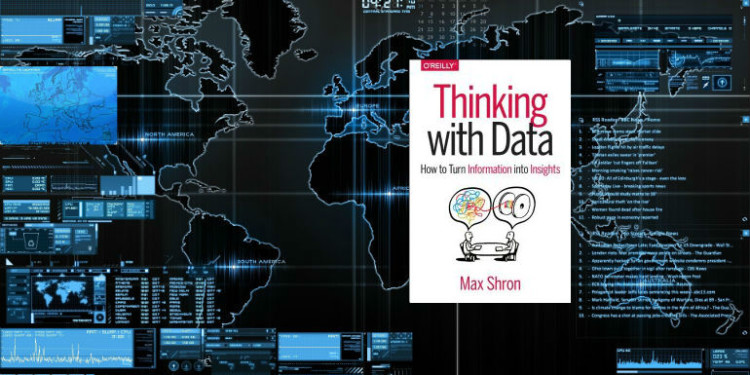
To be successful with data projects requires telling a captivating story at the beginning and concluding with actionable insights. Understanding goals, outcomes and narrative structure are key skills.
Thinking with Data: How to Turn Information into Insights by Max Shron
Although Thinking with Data is about data science, the approach author Max Shron provides has many parallels and applicable ideas for product development. Product managers must present customer and product data to inform design decisions and guide roadmap investment. Thinking with Data provides a detailed framework for framing data projects and linking each element for a compelling presentation. It’s divided into two parts: how to tell a good story about a data project and how to make good points with the data. The former enables project success by bringing clarity to the problem and objectives while the latter illustrates how to present data to provide knowledge for action. The book contains a number of examples and case studies that make the concepts tangible.
Shron devotes a large chapter to scoping data projects, cautioning the reader against just diving into data without thinking about the goals and desired outcomes. Establishing a context, audience needs, vision, and how the organization or team will manage and maintain the outcome is a great way to build a foundation for telling a story with data. This framework provides the setup, journey, and ending for the narrative of the data project. Arguments are the logical connections between the data itself, the questions being asked, and the ultimate conclusions.
To walk the path of creating things of lasting value, we have to understand elements as diverse as the needs of the people we’re working with, the shape that the work will take, the structure of the arguments we make, and the process of what happens after we “finish.”
Project Scoping
Starting with data, without first doing a lot of thinking, without having any structure is a short road to simple questions and unsurprising results. We don’t want unsurprising–we want knowledge.
Before embarking on a data project, it’s essential to think through the problem domain, goals, and future state so that you end up with knowledge instead of more data which does not impact your business. A project scope has four parts: context, needs, vision, and outcome that provide a narrative frame. Shron calls this the “what before how” phase and gives a guiding acronym: CoNVO. The context provides the exposition of the story, the need is the conflict or forcing function, the resolution is the vision, and the happy ending is the outcome.
Context
The project is the “defining frame that is apart from the particular problems we are interested in solving.” In a few sentences, it answers:
- Who are the stakeholders in the project and who benefits?
- What are these groups trying to achieve?
- What work or goal will this project contribute to?
Much of the information required to develop a context comes from talking with the stakeholders and engaging in their communities.
Needs
If working with data begins as a design process, what are we designing? We are designing the steps to create knowledge.
Project needs identify the knowledge gaps that, when addressed, will further the goals of the project stakeholders. Identifying needs is an iterative process which reflects current understanding back to the groups whose needs are to be met by the project.
The best needs statements establish a relationship to a specific action that relies on good knowledge. “A good need informs an action rather than simply informing.” If there is no direct link between a need an action, try to find a strategic imperative or perspective that will be better informed or able to move forward more effectively.
At first, needs may be relatively high level, but before a project is started, needs must be elaborated through a further questioning process (e.g., the Ljan Five Whys or just go through a question generation process surrounding the need to get better definition). This process will also apply to the vision.
Shron says that unless needs are well defined, the project is a high risk of producing “fluff”. He warns against identifying dashboards as needs and says “nobody except car drivers needs a dashboard.”
A data science need is a problem that can be solved with knowledge, not a lack of a particular tool.
Vision
The project vision is a sketch of “what it will look like to meet the need with data.” The format of the vision can be a simple graph that represents the desired knowledge to be gained. In addition to a visual or verbal depiction of the vision, an argument sketch establishes what is required to convince the audience at the end of the project.
One example Shron gives in the book is an anti-corruption campaign:
Vision: The developers working on the corruption program will get software that uses social media feeds to determine whether a political figure is getting media coverage. Program staff will receive access to a dashboard and get e-mail alerts.
Mockup: Politician X has been cited in several news talk show feeds.
Argument sketch: Since the system is correctly keeping track of a list of politicians, the anti-corruption program team has confidence in the monitoring service.
Outcome
The outcome is distince from the vision; the vision is focused on what form the work will take at the end, while the outcome is focused on what will happen when we are ‘done”
The outcome is the last mile of the project where the deliverables are now available to the organization and are effectively operationalized. The outcome must answer:
- How will the knowledge that is delivered by the project be distributed to and integrated within the organization?
- Who will use it and how will it be used?
- How will success be measured?
Key questions to establish the outcome are: who will manage the project deliverables next?; who or what will ensure that the project deliverables remain relevant and useful?; what will change after the project is complete?
As in any creative field, working with data is not a linear process where we proceed from a grand statement of the problem at hand and gradually fill in the pieces until we are satisfied.
Arguments
An argument moves from statements that the audience already believes to statements they do not yet believe.
The last chapters of the book outline how arguments are made. Since arguments are how the data is presented to achieve the project vision, they are a critical part of the project. Arguments contain claims which are typically backed by available data which has to be put into an intermediary, compact format to make it digestible within an argument.
Data science is the application of math and computers to solve problems of knowledge creation; and to create knowledge, we have to show how what is already known and what is already plausible can be marshaled to make new ideas believable.
Shron elaborates several aspects of arguments: the biases and beliefs that audiences have, what can be used as evidence and proof, patterns of reasoning that can be used to make an argument, types of disputes that can be used against an argument, and the dynamics of causation.
Before beginning a line of reasoning (argument), it is important to understand where the audience stands initially. An audience will have some preconceived notions, biases, and knowledge about the topic. Once the audience parameters are taken into consideration, an argument typically begins by making a claim based on evidence with a justification. Claims and sub-claims must be substantiated with evidence (data) and justification (linking the claim with the data through a table, chart, map, or other means).
With those basic building blocks, the argument can now be laid out according to several patterns of reasoning. The first pattern Shron lays out are categories of disputes that can take place in an argument. Facts, definitions, and values related to the argument can be disputed so the presenter needs to be prepared to counter this type of feedback from the audience. The argument itself can proceed from specific to general, or vice versa. Analogies can also be used to make a point as well as cost/benefit.
An important consideration in arguments is causation. Since there are no tools to establish causation, human reasoning must be used. Causal arguments exist in a spectrum, from loosely associated grouping to models which support high confidence predictability. Shron covers causality extensively and points out that when establishing a causal relationship the most important factor is identifying and ideally removing confounding factors which make causes and effects appear to be related when they are not.